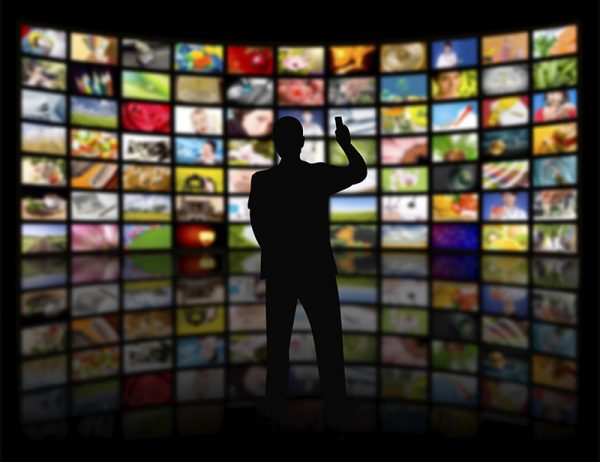
How wrong the polls were. After Donald Trump stunned the world by winning the White House this week, one common sentiment is how “off” the numbers were in gauging the ground sentiment.
Going into the big day, the real estate mogul had between a 1 and 30 per cent chance of winning, according to a good number of polls in the United States. Yet, he staged one of the most shocking of upsets.
One reason thrown up now is that this was an unusual or outlier of an election. Perhaps it was a “black swan” event that was nearly impossible to predict. Nothing about Trump’s campaign was usual, you could argue.
Yet another reason could be that the polls were not representative. Many groups that came out to vote this time, for example, did not do so before, so they were not taken into account when pollsters weighted their findings.
Whatever the reason, one thing is clear – the numbers don’t match the result. Either the data was not analysed correctly or everyone got the wrong data in the mix.
For Hillary Clinton, the result was devastating. Based on what the data told them, her campaign may not have paid as much attention to places where she believed she was clearly ahead, such as in Democratic strongholds like Pennsylvania, which she eventually lost.
This is also a wake-up call for many others who have been pushing for data – not gut feel, intuition or experience – to be the biggest factor in making important decisions.
At meetings in boardrooms everywhere, data has been trotted out as a means to win arguments. It has been used to chart an organisation’s direction, from how Netflix displays its content on your TV to how a government rolls out e-services to citizens.
Unfortunately, if you have the wrong analysis from your data, you make a fatefully wrong decision. Data-led or data-driven management may be trendy now, but it has issues.
For one, data usually gives you a probability, not an flawless prediction, of what may happen. Many adherents of new data models, however, are so certain they believe things are deterministic.
Interestingly, Trump’s camp reportedly had a data model that predicted a win for him. This contradicted what the majority of polls found.
Hindsight is easy, sure. The important thing is not that one camp was right, while the other wasn’t. It’s that there are several outcomes that can be predicted from various pools of data.
In other words, there isn’t a sure-fire way of making the right decisions based solely or mainly on data. For many people seeking absolutism in the ongoing data revolution, the Trump win will have shaken their faith in the newfound religion.
The many “ifs” and “buts” mean that clear-eyed human judgement is much needed. In the still-imperfect science of data analytics, there remain lot of uncertainties and variables that require an expert to check, verify and analyse.
While machine learning has enabled computers to better see patterns and identify outliers, the technology often still needs people to find and input the data – the right kind. As the saying goes, rubbish in, rubbish out.
When the experts get things right, they are lauded as geniuses, like the folks behind President Barack Obama’s campaigns in the past. When they fail, as with Clinton this week, they are said to have taken their eyes off some important things.
Between the two extremes, however, is the reality that data isn’t always right. Nor is it an infallible source of wisdom to base important decisions on. And even when everything works, sometimes you can’t change the outcome.
Clinton seemed destined to be her country’s first woman president. In the end, more women backed Trump, a man who had boasted about being able to molest women because he was rich. Analyse that.