Brought to you by Keysight Technologies
By Silviu Tuca
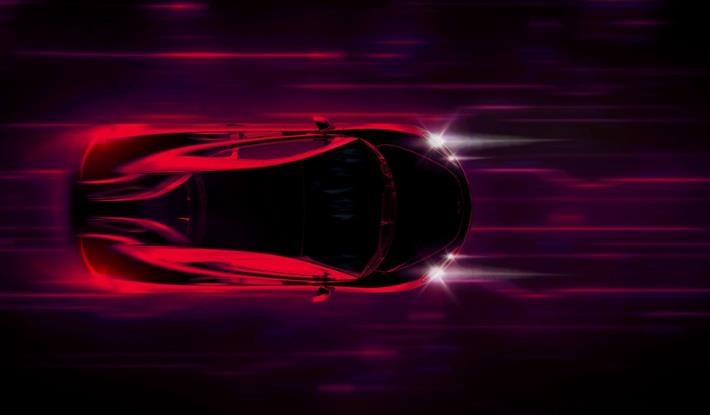
Creating safe and robust automated driving systems for future vehicles is a complex task. There are immediate challenges that automakers must overcome to realise the future of autonomous mobility.
Autonomous vehicles have hundreds of sensors, that all need to work in concert within the car and with other smart vehicles in their surrounding environment. The software algorithms enabling autonomous driving features will ultimately need to synthesise all the information collected from these sensors to ensure the vehicle responds appropriately.
The vision of fully autonomous vehicles is looming and along with improving the overall efficiency of transportation systems, driver and passenger safety is the most compelling advantage of self-driving vehicles. The most recent data suggests self-driving cars could reduce traffic deaths by as much as 90 percent (figure 1).
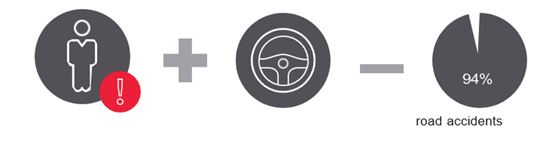
Level up vehicle autonomy
Advanced driver assistance systems in production vehicles have reached levels two and three, which in most traffic situations, require the driver to control the vehicle.
Many original equipment manufacturers (OEM) and industry experts believe pushing further toward levels four and five autonomy – where five represents vehicles not requiring any human interaction – will make our roadways safer (figure 2).
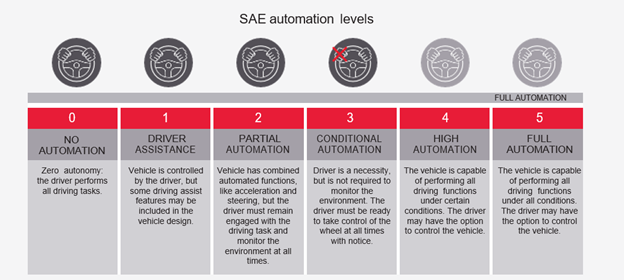
To achieve the next level in vehicle autonomy, many advancements are required. There will be massive investments in sensor technologies, such as radar, lidar, and camera which will continue to improve environmental scanning.
As each sensor type has its own advantages and disadvantages, they need to complement each other to ensure the object detection process has the required built-in redundancy.
Huge investments in computationally powerful software algorithms are also necessary to combine and carry the large amount of high-resolution sensor data including vehicle-to-everything (V2X) communication inputs.
Machine learning (ML) is the established method for training self-improving algorithms and artificial intelligence (AI). Those algorithms are then making decisions to ensure safety in complex traffic situations. Training these algorithms with the most realistic stimuli available, in a repeatable and controlled fashion in the lab, is crucial for their accuracy and their safe deployment.
The gap between roadway and software simulation testing
Today, a large amount of testing time is spent focused on sensors and their control modules (ECUs) by simulating environments in software or software-in-the-loop (SIL) testing.
Road testing of the completely integrated system within a prototype or road-legal vehicle allows OEMs to validate the final product before bringing it to market. Recreating a virtual world in the lab, with accurate rendering of the scenes, plus real radar sensors and signals, will bridge the gap between simulation and road testing.
The challenge today is the emulation of full radar scenes, especially when the scenes are complex and have many variables. The goal is to thoroughly test in the lab all driving scenarios, even the corner cases, before bringing the vehicle to the test track or open roadways.
Software simulation is used in the early development cycle. Simulation is possible of underlying sensors, vehicle dynamics, and weather conditions. Is that all it takes? Is it good enough to confirm what has been tested in plain simulation can now be taken to the real world? The software is ultimately an abstract view and it has imperfections.
Relying only on real-world road testing is also unrealistic because it would take millions of meters for vehicles to become safely reliable to navigate in urban and rural roadways 100 per cent of the time. To truly test the AV/ADAS functionality, it is necessary to control all relevant parameters.
To close the gap between real-world testing and simulation, real and physical sensors are needed in the test setup. This complexity must be added to the test to predict how AVs will behave on the road.
Under any circumstance, the vision is for technology to fully replace the human behind the wheel to enable reliable, accurate, and safe decisions on the road. Software simulation cannot fully test the real sensor response and testing on the track is not repeatable.
Today, specifically when emulating radar targets, there are several technology gaps.
Limited number of targets and field of view
A common approach ties each simulated target to a delay line. Even if additional targets are added, only one radar echo is processed at a time. Also, if an antenna array is created, it isn’t possible to simultaneously emulate targets at the extreme ends of the radar module’s field of view.
In addition, each movement of the antennas introduces a change in the echo’s angle of arrival (AoA), which might lead to errors and loss of accuracy in rendering targets, if not recalculated.
Inability to generate objects at distances of less than 4 meters
Many test cases, such as the New Car Assessment Program’s (NCAP) Vulnerable Road User Protection – AEB Pedestrian, require object emulation very close to the radar unit. Most of the target simulation solutions existing on the market today are designed for long distances.
Lower resolution between objects
Up until now, target simulators could only process one object as one radar signature – this leaves gaps in scene details.
For example, on a crowded multi-lane boulevard, test equipment must accurately tell the difference between all the traffic participants. With only one echo per object, the algorithm might not be able to tell the difference between a bicycle and a lamp post.
New technology is needed
Full-scene emulation in the lab is key to developing the robust radar sensors and algorithms needed to realize ADAS capabilities on the path to full vehicle autonomy.
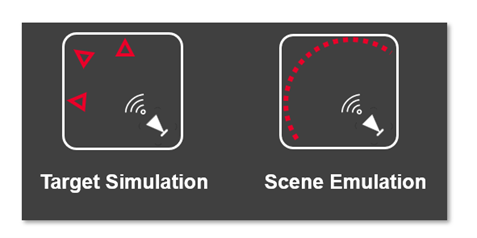
One method is to shift from an approach centered on object detection via target simulation to traffic scene emulation (figure 3).
This will enable the ability to emulate complex scenarios, including coexisting high-resolution objects, with a wide field of view and a reduced minimum object distance.
The sensor’s entire FOV must be covered to achieve high test coverage and run comprehensive test scenarios. A wide FOV is needed, ideally with RF front ends that are static in space, to enable reproducible and accurate AoA validation.
Realistic traffic scenes require the emulation of objects very close to the radar unit. For example, at a stoplight where cars are no more than two meters apart, bikes might move into the lane or pedestrians might suddenly cross the road. Passing this test is critical for the safety features of an ADAS/AD.
Object separation, the ability to distinguish between obstacles on the road, is another test area for a smoother and faster transition to level four and five vehicles. For example, a radar detection algorithm will need to differentiate between a guard rail and a pedestrian while the car is driving on a highway.
Achieve greater confidence in ADAS functionality
More targets, shorter minimum distance, higher resolution, and a continuous field of view are essential to real world testing. In the lab, this will enable an increase in test coverage to not only save time, but safely run and repeat test scenarios.
A traditional radar target simulator (RTS) will return one reflection independent of distance while a radar scene emulator increases the number of reflections as the vehicle gets closer, also known as dynamic resolution. This means the number of objects varies with the distance of the object.
AD and ADAS software decisions must be based on the complete picture, not only on what the test equipment allows. New radar emulation technology recently introduced from Keysight is one more way to shift testing of complex driving scenarios from the road to the lab. To learn more, please visit https://www.keysight.com/find/DiscoverRSE.
About the Author
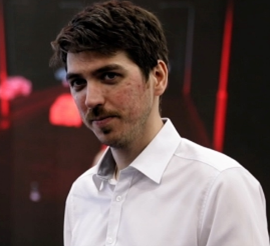
Silviu Tuca is the radar-based autonomous vehicle product line manager for Keysight Technologies. After obtaining an EE master’s degree in RF electronics and a PhD in Biophysics, he has spent his professional life working with test and measurement equipment – developing new calibration methods, technical consulting, or articulating the value of those instruments. Silviu is in Stuttgart, Germany, and in his free time enjoys being outdoors, listening to audiobooks or podcasts, and a good philosophical discussion.