Brought to you by Keysight Technologies
By Renee Morad, Autonomous Vehicles Solutions Marketing Lead, Keysight Technologies
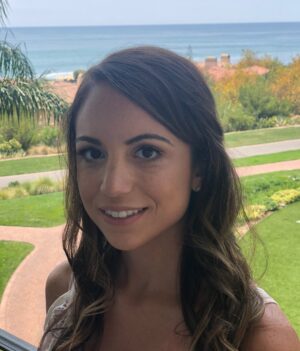
Seat adjusted. Check. Seatbelt fastened. Check. Ignition started. Check. Hang on tight; you’re in for the ride of a lifetime as your self-driving car races from zero to 192.2 mph—today’s record speed for an autonomous vehicle—on a futuristic test track.
As your self-driving car navigates this exhilarating course, decelerating for twists and turns, the stakes are high. That’s because on this test track, no variable in any given driving scenario is left unturned, and every variable is repeatably and reliably measured.
While you’re not driving the car, you’re driving the greater mission of this test run: to train your car’s autonomous driving algorithm so that it makes the optimal decision every time, with no catastrophic errors in the process.
This sounds like an impossible feat in the real world, where virtually every variable surrounding a self-driving car is unpredictable, and accidents during test runs are bound to happen.
However, a new fleet of digital twins are making these types of idealistic scenarios a reality in today’s digital test environments. While the concept of repeatably measuring every single variable around a self-driving car is still underway, we’re inching closer to this model with every new advancement in automotive emulation.
Automakers are increasingly enlisting the help of digital twins to accurately test and measure specific automotive components and features before they launch them in the real world, all while bringing innovation to market faster, safer, and more cost-effectively.
Digital twins are digital representations of real-time data and aid in building predictive models that help determine the probability of success of physical prototypes.
The global digital twin technology market, at US$9.5 billion today, is expected to increase at a compound annual growth rate of 22.6 per cent to reach US$77.65 billion by 2032, according to a recent report by market research firm Future Market Insights.
A variety of sectors, from healthcare and telecom to real estate and retail, are enlisting the help of digital twins, yet the largest market share of digital twin technology, at 15 per cent, comes from the transportation and automotive industry.
In the automotive industry, rising utilisation for simulation, designing, maintenance, repair, and overhaul (MRO), manufacturing, and car accident aftermath contribute to the need for digital twin technology.
This technology facilitates everything from testing complex safety scenarios for autonomous vehicles to gauging the importance of maintaining components of a race car engine at risk for getting damaged or burnt out, according to the report.
Using digital twin technology, automakers are now leveraging vast quantities of data in the lab collected from tests of self-driving cars to build complex simulations.
These simulations allow product developers to delve deeper into how an autonomous vehicle’s artificial intelligence (AI) will respond to unpredictable situations, such as weather conditions like hail or snow or a traffic jam. It also allows product developers to program a much wider set of functional tests in far less time.
For example, researchers can conduct crash-test simulations faster and more safely using digital twin technology. Self-driving cars, at a very basic level, are essentially robots operating in the real world.
Digital twins are the digital environments that humans can build, manipulate, and control to teach these autonomous vehicles how to safely operate in the virtual world before integrating their intelligence into real-world scenarios where they will interact with spontaneous events and human behaviours.
Today’s automakers are calling on digital twins for every automotive feature they test, from radar and C-V2X to in-vehicle network and cybersecurity.
By integrating multiple digital twins, they can build a comprehensive testing platform, where they can train the car’s autonomous driving algorithm to accurately see and react to its complex and dynamic environment.
Keysight’s Radar Scene Emulator, for example, provides full scene emulation of up to 512 objects at distances as close as 1.5 meters. By enabling radar sensors to see more with a wider, continuous field of view, it bolsters an algorithm’s training to detect and differentiate multiple objects in dense, complex scenes.
In turn, automakers can emulate real-world driving scenes in the lab with variations of traffic density, speed, distance, and total number of targets, moving up testing timelines for common to corner case scenes while minimising risks.
Designers are using digital twins to model sensors, test them against real-world scenarios in the lab, and explore new designs and sensor combinations. In addition, digital twins are being used to model a car’s in-vehicle network to test network bandwidth and data speed to improve reaction times.
When it comes to cybersecurity testing, automakers are calling on digital twins to test all access points in one design environment to produce a clearer picture of how secure a vehicle will be in the real world, while eliminating safety threats.
To be sure, using digital twin technology in automotive product development invites some challenges. Emulation, in general, can never completely represent the real world, but by adding, say, noise or stress to a testing environment, the digital twin can get quite close to replicating real-world scenarios.
On the flip side, because the variables are not completely random, product developers can control and manipulate these variables, increasing the likelihood to get it right faster and minimising costly rework and schedule delays.
What does this mean for the future of automotive innovation? Let’s echo what we said before: we’re in for the ride of a lifetime.
By essentially bringing the road to the lab, the automotive industry is undergoing a paradigm shift for innovation—and digital twins are the quiet but powerful forces enabling these new possibilities behind the scenes.
Renee Morad is the autonomous vehicles solutions marketing lead for Keysight Technologies. Prior to joining Keysight, she has worked as a writer and editor for more than 15 years. She has been published in the New York Times, Scientific American, NBC News, Forbes, SmartMoney, the Wall Street Journal Magazine, and other outlets. She has also written marketing content for companies such as Dell, HP, Microsoft, Audi, JPMorgan Chase & Co., and others. Renee graduated from New York University, where she studied journalism and creative writing.