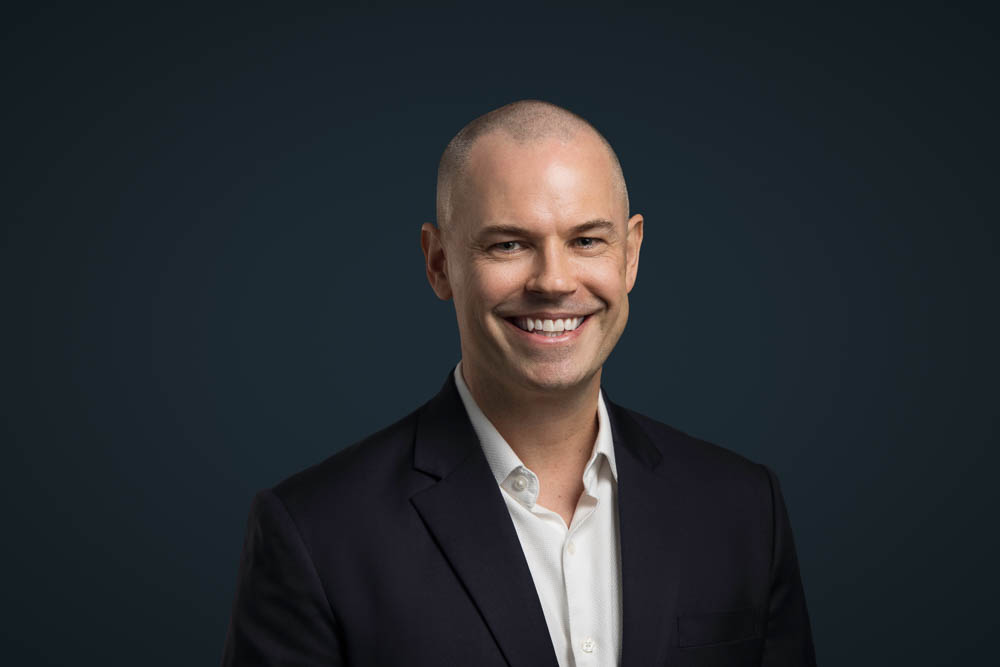
Organisations in the Asia-Pacific are using or plan to use AI applications as a way to keep ahead of the technological curve.
Some 88 per cent of Asia-Pacific organisations are using or plan to use AI applications in the next 12 months, according to IDC. Generative AI, in particular, is growing in popularity, with nearly two-thirds of organisations investing in or planning to invest in it by 2023.
Businesses in the region are working to protect sensitive information and to safely and economically get value from Large Language Models (LLM). LLMs are being used for everything from improving developer efficiency, to providing analysts with summaries of complex dense reports and improving the efficiency and effectiveness of customer call centres.
Usage policies are being carefully developed and self-hosted LLMs are increasingly being deployed to complement the use of SaaS-based LLMs. There is also a focus on ethical and responsible AI with governments and regulatory bodies playing an increasingly important role.
Regulatory bodies now are under pressure to address issues around data privacy and security, intellectual property rights, and the potential misuse of AI-generated content, with countries like India are drafting the Digital India bill to regulate AI and keep its digital citizens safe.
Singapore has launched its AI Verify Foundation to promote the development of tools for responsible AI usage, and boost AI testing capabilities to meet the needs of companies and regulators globally.
With this nascent technology, organisations have to consider key risks and limitations of AI today, even as they pursue its benefits, says Daniel Hand, field chief technology officer for APJ at Cloudera, which provides data analysis tools for cloud-based data.
In this month’s Q&A, he calls for organisations to better understand the risks of AI and consider how to carefully innovate for successful AI implementations.
NOTE: Responses have been edited for style and clarity.
Q: What are some of the key risks and limitations of AI today, in terms of enterprise use?
A: Ethical issues (especially bias and discrimination), data privacy, data security, transparency and explainability, and concerns around the accuracy and relevance of answers are significant risks associated with AI models.
These risks can impact an organisation’s brand and service reputation. A larger, contextually-relevant training dataset leads to better outcomes, but suboptimal or misleading results may occur if suitable context to sensitive data or if data lineage is questionable.
AI models can be influenced by bias, often due to poor data preparation during the model training process. This can result in negative outcomes like lost service or revenue, and legal consequences. There have been several high-profile cases of bias influencing credit limits and insurance policies.
AI-supported decisions made within an opaque black box where there is a lack of explainability and transparency can introduce risks that may violate industry guidelines and data protection regulations.
An example is the dismissal of workers in the Netherlands without either suitable human intervention or transparency and explainability in AI supported processes. The employer was found to have violated article 22 of General Data Protection Regulation (GDPR).
There have been significant advances in AI and ML algorithms and in the performance of LLMs. However, few organisations have the resources to train these models. They can either consume closed-source proprietary models as public SaaS services or host Open Source models in a trusted environment.
The risks include a lack of transparency, biases, and sharing incorrect information or worse, sensitive data. Some reported cases have led to the organisations tightening usage policies, often with a blanket ban on using public SaaS-based LLMs.
Plus, generative AI models often lack contextual understanding of enterprise questions, leading to incorrect or inaccurate responses.
For example, a chatbot replying to a query on warranty duration can fail to provide important context. That causes confusion and misunderstanding, especially when dealing with issues outside warranty coverage. This can negatively impact customer satisfaction, credibility, and trust in the business.
Q: What can organisations do to mitigate such risks?
A: Let’s focus on the risks of data privacy, contextual-related performance, and ethical or responsible AI.
Data privacy risks are crucial for organisations to mitigate. To ensure data privacy, organisations should classify data and provide clear guidelines on usage. For instance, using a SaaS-based LLM for sensitive internal documents may violate data management policies.
Besides putting in place policies, guidelines, and technology to control data privacy, organisations need to augment SaaS-based solutions with their own privately hosted solutions that provide comparable performance.
To ensure contextual relevance and performance, organisations should control access to the prompt and inject relevant context through Retrial Augmented Generation (RAG).
Responsible or ethical AI is multifaceted, with bias being a significant element. To address bias, organizations should understand the bias in the training data and in-built biases in pre-trained models. Connecting with governing bodies within their industry, such as Monetary Authority of Singapore (MAS) for financial institutions in Singapore, is recommended.
There are two main approaches to benefiting from LLMs: Public SaaS-based LLMs and privately-hosted LLMs based on open source models. A combination of data sensitivity and economic efficiency determines whether it’s appropriate to consume SaaS-based LLMs.
Achieving trusted data across the entire data lifecycle across public and private clouds is essential for ensuring trusted data and broader AI and ML use cases.
Q. What are some best practices for organisations to take note of when adopting enterprise AI?
A: I would start with a clear usage policy, strong data management controls, and a scalable, reliable approach to machine learning operations (MLOps). These are crucial for analytical use cases like data warehousing and predictive analytics. AI models, particularly ML and Deep Learning, perform better with high-quality data.
Next, data ethics and responsible AI should be influenced by relevant industry bodies.
Organisations should have clear data usage policies, which require classification and approval of data, algorithms, models, and services. Regular training and updates are essential for personnel to understand licensing and fair usage policies.
For example, SaaS-based developer productivity services may be restricted to only a subset of non-sensitive development projects. However, with the introduction of a privately hosted LLM based on the Open Source StarCoder LLM, the policy is extended to include this new capability for sensitive development projects.
Finally, most AI models struggle to get out of the lab and into production efficiently and at scale. One solution is Machine Learning Operations (MLOps) which covers everything for data exploration, data engineering, model training, model tuning and subsequently making those models available for consumption.
It also includes the process of monitoring model performance and retraining models when appropriate with suitable human oversight.
Q. What are enterprises in Asia-Pacific doing to prepare their business to be “AI-ready”?
A: Organisations in the Asia-Pacific are focusing on data management, data platform capabilities, and AI-readiness.
An example is OCBC bank, which has developed strong data management and platform capabilities, such as integrating LLMs into their on-premises business.
They have successfully replaced existing developer code assist tools with privately-hosted services based on StarCoder LLM. This has reduced operating costs and enhanced the service to be more contextually specific with their own coding standards.
Besides a strong technology capability, building strong data science and data engineering skills is essential to take advantage of the available algorithms and models.