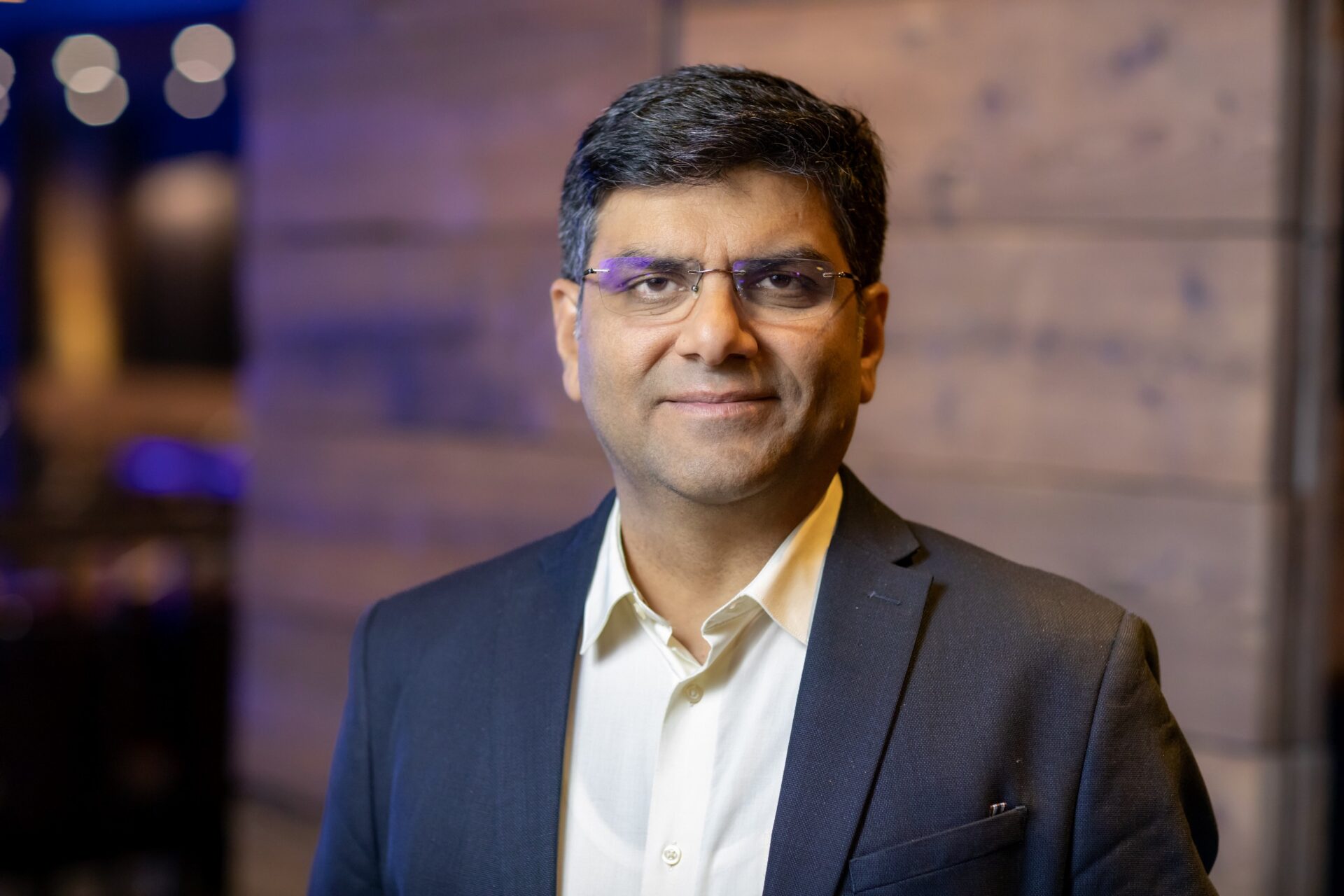
So much of today’s modern digitals services, from ride hailing to stock trades, depend on real-time data streams that are generated from thousands of sources and processed on the fly.
Yet, this so-called data streaming trend is only just beginning, say experts, who add that a lot more sensors, users and other data sources will come online.
Fraud detection, for one, will be an important use case for data streaming because the huge amounts of data a bank collects can help it better detect anomalies, says Suvig Sharma, area vice-president for Asean at Confluent, a company that provides data streaming solutions.
And with AI becoming an everyday tool in the workplace, the ability to analyse and make use of real-time data will be important for business, he tells Techgoondu, in this month’s Q&A.
NOTE: Responses have been edited for brevity and style.
Q: What applications and use cases have been driving data streaming in Asia-Pacific in recent years?
A: With every industry reliant on real-time data, today, data streaming offers endless opportunities. This includes enabling organisations to power personalised consumer experiences, strengthen cybersecurity measures and ensure proper data governance.
In Singapore, we work with Trust Bank to enable its cloud-native data architecture, allowing the digital bank to power unique, secure and real-time experiences for customers at both scale and speed.
Confluent’s data streaming platform also provides Trust Bank with built-in governance to meet regulatory requirements and enhance customer trust.
By connecting data pipelines across the bank, we help eliminate data silos and allow teams to share data at customisable levels of access control, reinventing the digital banking experience for their customers.
We have also worked with regional telco Globe Group, to maximise the use of their real-time data. We helped Globe transition from batch-based to real-time processing, allowing them to offer improved, personalised marketing campaigns, achieve better platform reliability as well as reduce infrastructure and manpower costs.
Data streaming is also crucial for businesses to detect and mitigate fraud and cybersecurity threats in real-time. The processing of large volumes of data across multiple sources instantly provides companies with contextual intelligence for end-to-end threat analytics, enabling them to detect anomalies as they occur.
As online fraud becomes increasingly sophisticated, data streaming used in combination with machine learning can help organisations assess potential threats and trigger early warnings, mitigating significant revenue loss and damage to brand reputation.
Q: There’s a huge expectation of live or real-time insights today with faster bandwidth and higher compute performance on the edge, but do we need real-time data analysis all the time?
A: The goal of processing data in real time should not be seen as just a tool to get “faster” results but as a form of re-thinking business processes entirely. Compared to traditional batch processing, data streaming allows businesses to become event-driven, or reacting to business events instantaneously as they occur.
From stock trades to retail inventory management and digital payments, they all require real-time data to provide unique context and extract the most relevant information for precise insights and data-driven decision making across the organisation.
Real-time data is the very foundation for building the next generation of faster, scalable infrastructure that is essential for companies to stay competitive and agile in today’s economy.
Q: As we know, cloud costs are ratcheting up for many businesses. Do you see some analysis workloads being put back on batch processing instead of being run in real time?
A: Batch processing actually requires the same amount of computational power as real-time data streaming. However, the key difference, and one that determines cost-efficiency, lies in the amount of storage required and the flexibility to scale.
This is where real-time processing provides a huge advantage over batch processing due to the distributed systems used in its infrastructure.
For example, retail companies may face higher volume of transactions during holiday sales, but a lower volume during off-peak season. When this happens, companies using stream processing can scale up or down to using containerised deployment, allowing them to stay efficient in managing their inventories and resources.
On the other hand, batch processing systems are usually housed in servers sized for maximum transaction volume, with a fixed headroom allowance. It thus generally ends up being more costly to sustain computational power for batch processing systems.
A scalable data streaming platform is the preferred choice for providing enterprises with the real-time data insights they require to stay ahead, while ensuring flexibility and cost efficiency according to their business needs.
Q: How does AI change the equation in the years ahead?
A: Data becomes information when context is applied and it is re-oriented for human consumption. The ability to pull real-time data into AI systems opens the door for new correlations and connections to be made.
For AI to be effective, its algorithms require continuous data ingestion. This allows for the system to integrate and overlay existing knowledge with new information, ensuring a rich context is available for AI training models to operate in.
Data then, as we can see, plays a vital role, and organisations need to ensure that the data fed into AI models must be well-governed, trusted and up-to-date. Data streaming can help to address data accessibility by aggregating multiple data sets from different domains, for companies to effectively leverage AI for actionable intelligence.
With a real-time data mesh, data can be democratised across organisations and AI teams are able to tap into data in a frictionless manner to power their models. This is infinitely more efficient than point-to-point integrations that eventually bottlenecks entire processes that result in increased backend complexity and resources.
Despite the tremendous impact data accessibility can have in conjunction with AI, there is still significant responsibility to ensure that data is properly governed and protected from misuse. This is especially important in the context where customer data is used.
A data streaming architecture by Confluent provides granular levels of role-based access control (RBAC) and attribute-based access control (ABAC), empowering these frameworks with a responsive, secure, and scalable approach for transforming how data can be used to work smarter with AI.