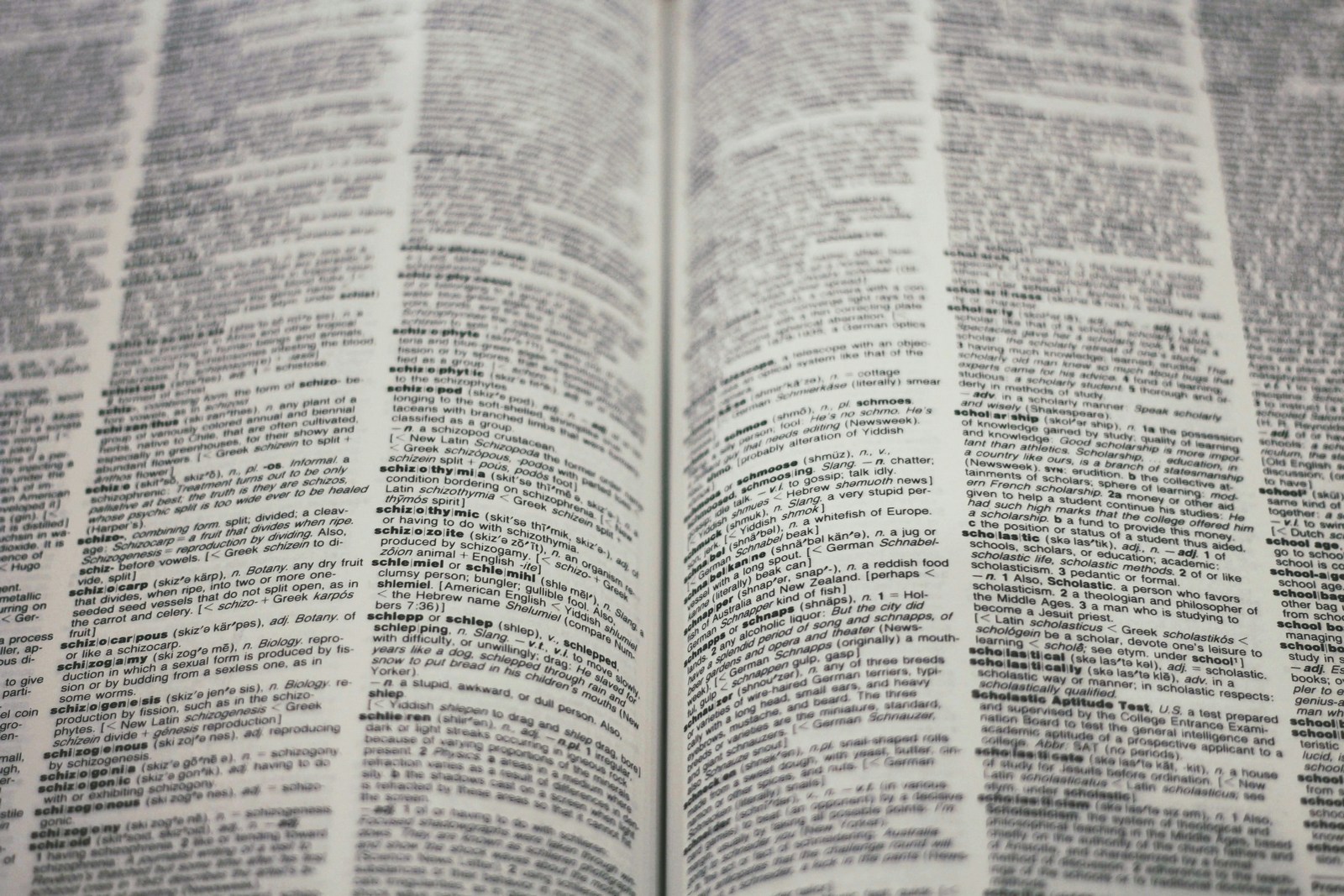
Large language models (LLMs) may impress with their ability to perform a wide range of tasks with great efficiency. However, small language models (SLMs) are emerging as a significant and practical alternative, say industry experts.
SLMs are optimised for lower-resource environments, they say, thus requiring less computational power and fewer resources compared to LLMs, which boast billions of parameters. This makes SLMs ideal for delivering high performance for particular applications without the need for extensive infrastructure.
According to an article in the scientific journal Nature, LLMs require volumes of expensive computing resources from graphics processing units (GPUs). The article also noted that a generative AI-driven search uses four to five times the energy compared to a conventional web search.
Kalyan Madala, IBM Technology’s APAC Software pre-sales engineering leader explained that SLMs require far less computational power and resources as they can operate locally on everyday hardware like laptops, mobile phones and edge devices.
This on-device processing, especially in edge computing and Internet of Things (IoT) applications, enhances security as it reduces data exposure and the risk of unauthorised access.
Advantages of SLMs
As SLMs have a simpler architecture and use smaller datasets for training, it makes them more explainable than LLMs – allowing humans to better understand and trust the output generated.
“The models are simpler and more interpretable, boosting transparency and aiding adoption. This AI explanability is essential for building trust in sectors like law, finance, and healthcare,” said Madala.
“Without the necessity for costly, specialised infrastructure, SLMs give smaller businesses and startups a cost-effective solution that doesn’t sacrifice effectiveness or versatility, which is crucial for real-time applications and scenarios where latency is a concern,” he added.
In Asia-Pacific, SLM adoption is driven by resource constraints, as many emerging markets in the region have limited access to high-end computational resources, he suggested.
SLMs can be fine-tuned to support local languages and dialects, suitable for a linguistically diverse region like the Asia-Pacific. SLMs can also support diverse applications, from enhancing customer service in retail to automating tasks in manufacturing.
“For users, what’s important is not just the size of the model but having the choice to customise and tailor their foundation models for their evolving use cases,” said Madala.
“Organisations should also have the flexibility to deploy the model in the infrastructure of their choice, depending on the use case and operational considerations,” he added.
“AI guardrails and continuous monitoring ensure that model deployments are secure and reliable as organisations scale up generative AI applications,” he stressed.
The emergence of niftier models
IBM recently launched the open-source Mistral AI Model on the watsonx platform – a compact LLM that is touted to require less resources to run, but is as effective and has better performance compared to traditional LLMs.
Other vendors have announced smaller AI models this year, including Microsoft and Google. For example, Microsoft has revealed Phi-3, its series of OpenAI SLMs that are smaller and less compute-intensive for generative AI solutions.
In February, Google unveiled Gemma, a series of lightweight open source generative AI models designed mainly for developers and researchers. According to Google, these models can run on laptop or desktop computers.
In terms of regional trends, most LLMs today are from the United States (73 per cent) and China (15 per cent), according to research in 2023 by the Large European AI Models initiative.
Within the Asia-Pacific, China is the key producer of LLMs, with the only other country producing LLMs in the region being Singapore with three models, according to Stanford’s AI Index Report released in 2024.