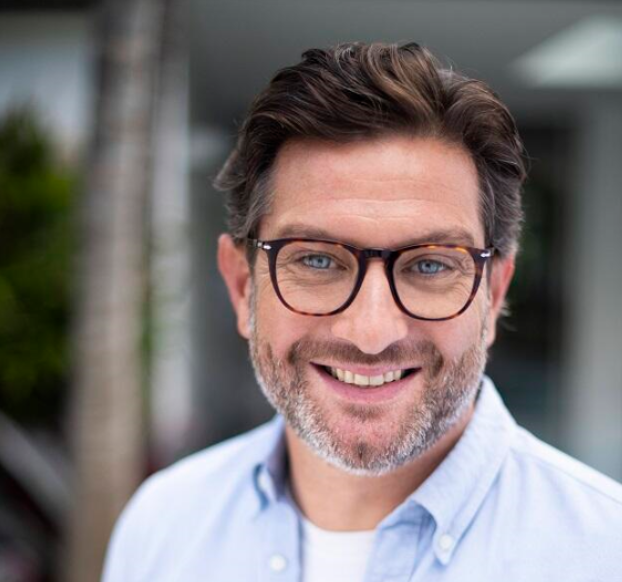
At a time when AI is often operating in a “black box” and its outputs are unquestionably accepted, a relatively nascent form of database technology may help untangle how it arrives at a result, say its proponents.
Graph databases focus on the nodes and relationships in a sea of data to reveal connections and insights not obvious with traditional databases.
By revealing such connections, graph databases can help make AI applications more accurate, explainable and transparent, says Kristen Pimpini, vice-president and general manager for Asia-Pacific at Neo4j.
For example generative AI (GenAI) chatbots can be more precise and detailed with training that taps on a knowledge graph of an organisation’s private data, he explains.
Neo4j’s graph technologies have been widely deployed for a while, for example, by banks to help identify relationships between various data points and identify possible fraud. Now, graph technologies could also enhance AI.
One way to do this is by presenting context, says Pimpini, in this month’s Q&A. “It helps to analyse data, spot patterns, and uncover misinformation.”
NOTE: Responses have been edited for clarity and style.
Q: We’ve had graph analytics and machine learning for a while now. What do the latest developments in AI bring to the table that wasn’t there before?
A: GenAI is the new best friend of graph analytics and machine learning. Combining GenAI models such as large language models (LLMs) with graph databases results in AI applications that are more accurate, explainable, and transparent.
A frustrating challenge facing companies right now is AI chatbots that don’t provide detailed and precise responses. This is where a machine-learning approach called RAG (Retrieval Augmented Generation) comes into play.
In contrast to LLMs that are trained on publicly available data, RAG taps into a Neo4j knowledge graph composed of your own private data to give LLMs more context, so the answers are improved and more personalised.
Furthermore, integrating Neo4j’s graph database with LLMs allows users to interact with knowledge graphs using natural language, making these tools accessible even to non-technical users. What used to be messy and unstructured data is now being transformed into knowledge graphs, that lead to deeper insights.
Neo4j’s graph algorithms and data science tools also enable advanced analytics and machine learning on graph data structures.
This allows organisations to use the predictive power of graphs to identify relationships and network patterns that can tackle complex challenges such as fraud detection, recommendation systems, and patient care management.
Q: When it comes to fraud, are people justifiably worried about impersonation through AI, such as the recent case in Hong Kong where an executive was fooled into transferring millions to fraudsters because he thought he had a video call from his CFO?
A: With AI advancements, deep fakes and fraud are on the rise, and it’s no surprise people are worried. Globally, fraud losses are expected to hit a staggering US$95.9 billion by 2027, and AI plays a big part in that.
In Singapore, deep fake cases jumped tenfold this year. The underlying problem is that deep fake tech is evolving so quickly that fraud-detection systems struggle to keep up.
Here’s where knowledge graphs shine through. By connecting data points to spot hidden patterns and anomalies, businesses can improve their fraud predictions. Even a small boost in fraud prediction accuracy can save companies millions.
For example, Neo4j teamed up with BNP Paribas Personal Finance to build a new fraud detection model. By switching from a relational to a graph database, they could spot loopholes between credit applications that fraudsters exploit, making real-time payments more secure.
Such efforts resulted in a 20 per cent drop in fraud, enabling automated fraud detection that approves or rejects applications in less than two seconds.
Q: Would your AI tools that help deter fraud today be able to defend against more advanced fraud attempts, say, through a fake voice or video call?
A: Traditional fraud detection tools just don’t cut it like they used to and prove to be a challenge in targeting today’s more sophisticated fraudsters.
These tools often miss connections between real-world entities and rely too much on historical data, making it tough to catch real-time fraud, especially when new behaviours pop up that weren’t in the old data.
While Neo4j isn’t specifically built to combat fraud, our graph technology is great at helping organisations spot and predict fraud by analysing connections between data points with greater speed and flexibility.
Companies get a much clearer picture of potential threats to link fraudulent activities more easily and accurately in real time. Graph databases can also store complex networks of transactions, accounts, and people, making it easier to detect perceived threats as they happen.
A useful example is the Panama Papers investigation. The International Consortium of Investigative Journalists (ICIJ) used Neo4j’s graph database to untangle complex networks of offshore accounts, exposing the key people behind companies hiding money in tax havens – including 140 politicians from over 50 countries.
Q: Seeing is no longer believing, as the saying now goes. Do you think AI will make a difference in a “post-truth” world where people already believe what they want to believe?
A: AI is being increasingly used to spread misinformation and create deepfakes, making it harder to tell what’s real and what’s not, and potentially manipulating public opinion.
It is a wake-up call for governments around the world to prioritise effective AI regulations. How AI shapes our post-truth world really depends on how ethically and responsibly we develop and use it. If done right, AI could be instrumental in restoring trust.
Graph technology promotes the evolution of AI by empowering the development of sophisticated AI applications by presenting context. It helps to analyse data, spot patterns, and uncover misinformation.
An example of this is the work Neo4j is doing with Syracuse University on election misinformation. Neo4j’s graph technology is helping to reveal hidden connections and interactions in a complicated network of social media content, identifying bad actors within a firehose of misinformation coming at voters ahead of the United States 2024 elections.