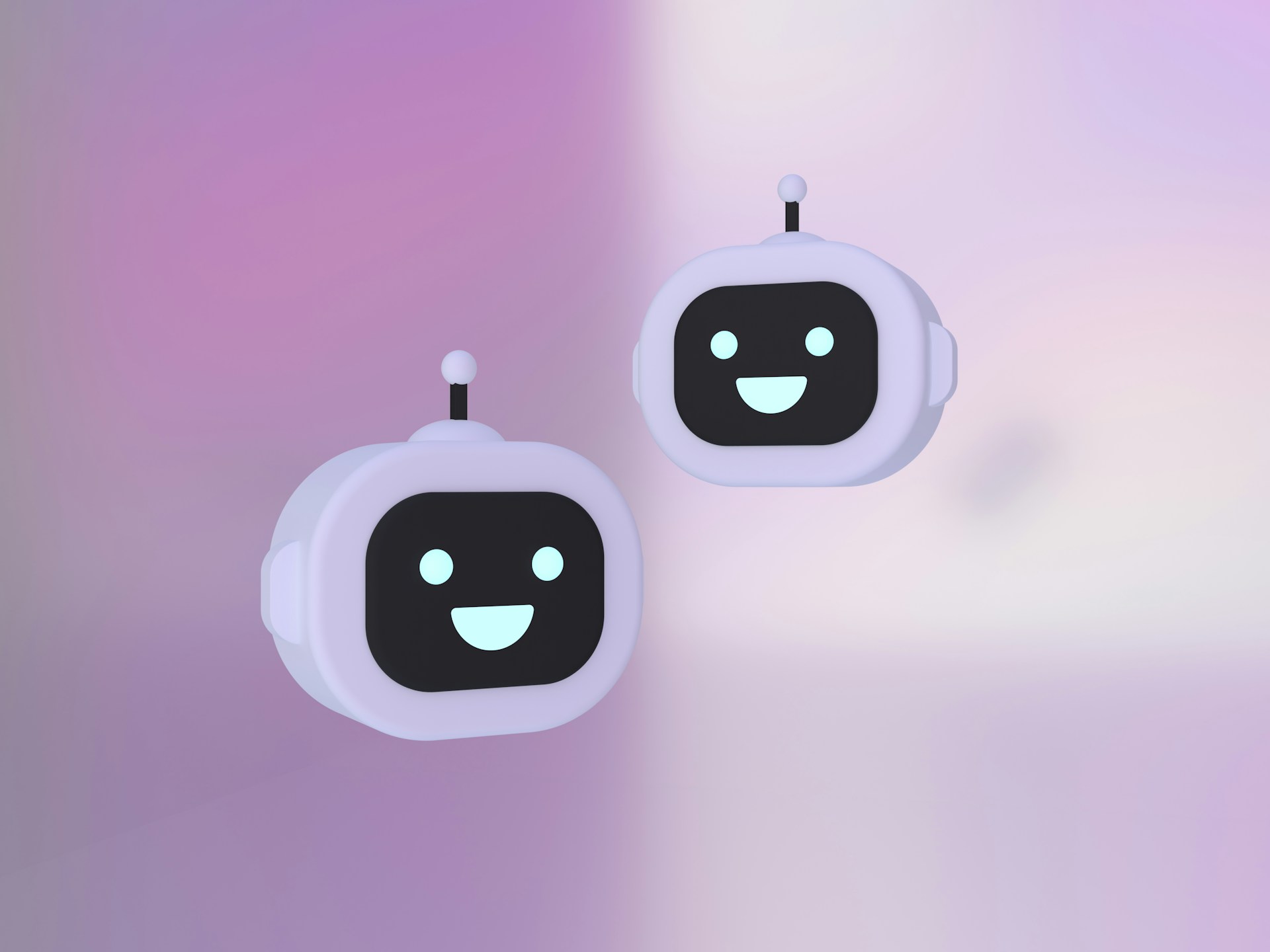
Businesses keen on making the most of large language models (LLMs) and generative AI (GenAI) have to first make sure they have a robust foundation, starting with a process of gathering data comprehensively and centralising it, according to consultancy firm Accenture.
After this, the data has to be “cleaned” rigorously over several months to ensure the model learns from accurate, relevant information, free from biases and inconsistencies, said Dr Ramine Tinati, managing director and Asia-Pacific lead for the Accenture Centre for Advanced AI.
Robust data infrastructure goes beyond just data cleansing; it requires clear guidelines on accountability for data accuracy, completeness, quality, usage, security, and governance, he said, adding that such measures are vital for building trust among users and customers.
“For organisations to achieve a meaningful data structure, they could use a pre-trained model like the Llama series, which they somewhat trust, and bolster it with their own organisational content to fine-tune or pre-train their model,” he stressed.
“To build meaningful data structures, organisations can leverage pre-trained models like the Llama series as a foundation, and bolster it with their own organisational content to fine-tune or pre-train their model to get accurate outcomes,” he noted.
Cleaning the data is a labour-intensive task that can take months, as it involves extracting potentially harmful and malicious elements. While tools like machine learning can automate parts of this process, human expertise is essential.
“You need people, usually data scientists, provide the human touch to make the model more relevant and efficient,” said Dr Tinati.
This process is important for transparency, as it spells out responsible data ownership, which is critical to engender trust for users and customers, he explained, in an interview with Techgoondu in conjunction with the setting up of Accenture’s AI Refinery Engineering Hub in Singapore last month.
He pointed out that a prevailing trend is for organisations to adopt a combination of LLMs and retrieval-augmented generation (RAG) systems. This approach is particularly appealing for businesses seeking basic information retrieval with a natural language interface.
“Most organisations want a natural language style system where you ask a question and get a response like chatbots. Thus, you need a bit of an LLM to allow you to interact in a more human-like form,” he said, stressing that this synergy between RAG architecture and LLMs facilitates more intuitive user interactions.
As AI technology becomes increasingly central to organisational strategies, cost considerations have also emerged as a significant concern. Training models can be expensive, requiring extensive computational and cloud resources due to the need for multiple iterations over large datasets and complex calculations.
In contrast, inferencing costs are lower since they involve applying a trained model to new data for predictions or outputs, which is far less computationally intensive.
However, what organisations truly care about is tokens per second (TPS) as a benchmark of cost efficiency. TPS has become a pivotal measure in evaluating the performance of LLMs due to its direct impact on throughput, user experience, scalability, and competitive advantage.
Dr. Tinati illustrated this point by stating: “So if it costs US$1,000 per hour to run the model and you get a million tokens out, that’s okay. If you’re getting 10 tokens, that’s probably not so good.”
Hence organisations must identify meaningful benchmarks that align with their operational goals to control costs, he added.
Another emerging trend is the adoption of hybrid cloud systems where part of the data resides in the cloud while another part is hosted on-premises.
This approach addresses compliance and security concerns which are emerging in Singapore, Indonesia, Australia and other countries, where governments impose strict information security regulations and data residency requirements.
“We are seeing a lot of investment in this region in such solutions,” Dr Tinati noted. “We have customers who use the cloud for non-sensitive workloads while keeping AI compute in their data centres to keep up with the tighter restrictions on data movement.”
Despite concerns about data scarcity, he does not believe that the world is running out of data. While much knowledge from Western sources may have been ingested into current LLMs, there remain vast amounts of untapped content in other languages such as Chinese and other non-Western languages.
Furthermore, he pointed out that video and visual–related data have been minimally captured thus far. “There’s plenty of data to go.”
The conversation around synthetic data also merits attention. Dr Tinati pointed out that organisations can leverage synthetic data in several ways.
First, when they lack sufficient real-world data to model specific scenarios—often due to consent issues or security constraints preventing certain datasets from being sent to the cloud. Second, for modelling and simulating real-world scenarios that are difficult to capture naturally such as black swan events.
For instance, predicting traffic flow during an unprecedented event like sudden congestion across all roads in Singapore requires generating synthetic data to forecast potential outcomes.
“We don’t have that data because it’s never happened,” said Dr Tinati. “So, you need to generate synthetic data to look what the outcomes would be.”
Accenture’s Centre for Advanced AI in Singapore is part of its global investment and efforts investment to advance the use of AI. It will offer a range of programmes designed to develop local talent and equip enterprises with the skills and knowledge needed to build AI applications responsibly.
Dr Tinati spoke to Techgoondu on December 3 after an Accenture panel discussion featuring its CEO and chairman Julie Sweet and Minister for Digital Development and Information Josephine Teo, where they spoke about the increasing importance of AI and the role of employers supporting women returning to the workforce.
Sweet was in Singapore in conjunction with the launch of the company’s Women Of Worth initiative, which will offer 10,000 women returning to the workforce free technology courses like GenAI, design thinking and project management. The initiative is in collaboration with the Singapore Business Federation (SBF).