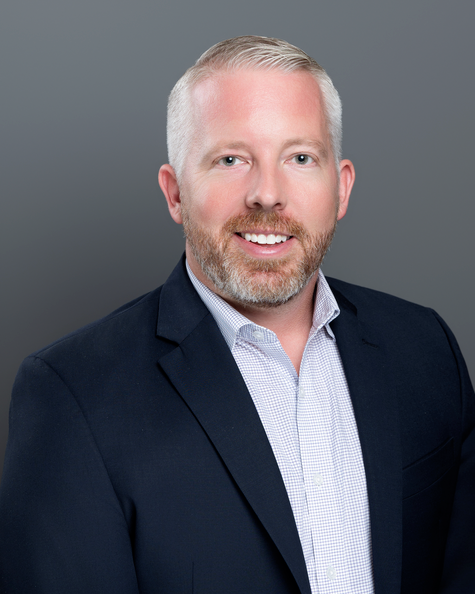
At a time when AI efforts are being hampered by real-world issues like data quality, Syniti is proposing that organisations use AI to improve the quality of the data that is used for AI itself. What could go wrong, right?
Well, the company that helps big businesses manage and migrate data is not just letting AI curate and process data for its own use, but to have human experts “join forces” with AI in an effort to create good-quality data that improves responses.
These experts with years of business experience “help procure and refine the high-quality data rules and reports that are ultimately used by our customers, ensuring alignment with real-world business processes and requirements,” says Cody David, GenAI managing solution architect at Syniti, which was acquired by consultancy firm Capgemini last year.
“This blend of human expertise and AI-driven analytics keeps organisations in control, prevents AI systems from going off track, and facilitates proper handling of critical decisions,” he adds.
This is important at a time when the industry is pushing ahead with AI agents that are expected to be semi-autonomous is finding problems and resolving them without much human intervention. However, all this is only possible with a good data foundation.
“From our conversations with various customers, it’s clear that data readiness varies widely,” says David. “Some organisations are reasonably prepared, but many still struggle to standardise and govern their data, which is critical for techniques like retrieval augmented generation (RAG)”.
“A single inaccurate data point can cascade into multiple downstream errors, so if you find yourself constantly repairing operational or analytical reports to keep basic business processes running, an AI agent that makes unsupervised decisions is likely premature,” he tells Techgoondu in this month’s Q&A.
NOTE: Responses have been edited for brevity and style.
Q: There’s been a lot of talk about agentic AI of late. What is the biggest breakthrough that it will bring for businesses in the next 12 to 18 months?
A: Agentic AI is evolving from systems that rely on hardcoded task lists to ones capable of sophisticated reasoning and planning. Over the next 12 to 18 months, the most significant breakthrough will be how these AI models handle the “planning” phase of complex business processes.
In the past, the likelihood of AI making even a single mistake – say, one out of 20 steps – was high enough to send an entire process down the wrong path. For truly effective agentic AI, we need near-perfect accuracy – 20 out of 20 steps correct.
Achieving this requires more robust reasoning methods, often through the integration of multiple models that can validate each other’s outputs. As these new planning approaches become more accurate and flexible, organisations will be able to develop AI-driven workflows that adapt dynamically to changing conditions, dramatically reducing the manual effort required to maintain and update AI-based automation systems.
However, do not be mistaken in thinking that it’s solely the responsibility of model creators to solve these challenges. The real-world problem-solving and application of these models is just as critical as the models themselves. One without the other is not a truly powerful solution.
Q: Many early AI trials have stumbled because of a lack of good data. From your conversations with customers, do you think most businesses have the quality of data needed for AI to run properly, say, to set up RAG to work with a large language model (LLM)?
A: In most enterprise use cases, particularly those that answer questions about data or take actions based on it, having consistent, high-quality information is essential. From our conversations with various customers, it’s clear that data readiness varies widely.
Some organisations are reasonably prepared, but many still struggle to standardise and govern their data, which is critical for techniques like RAG.
A single inaccurate data point can cascade into multiple downstream errors, so if you find yourself constantly repairing operational or analytical reports to keep basic business processes running, an AI agent that makes unsupervised decisions is likely premature.
Ensuring reliable data is a fundamental prerequisite before taking full advantage of advanced AI, including LLMs or agentic AI.
Q: How does AI-assisted data, as Syniti sees it, help improve the success rate of AI efforts?
A: Syniti views AI-assisted data through two complementary lenses: “data quality for AI” and “AI for data quality”. Data quality for AI emphasises the importance of accurate, trustworthy data to power chat assistants or agentic AI systems. If the underlying information is flawed, any AI-driven automation or insights will also be suspect.
On the flip side, AI for data quality focuses on using AI to detect issues, fix inaccuracies, and enrich data to make it more complete. AI-driven processes can streamline data standardisation, matching, and enrichment, activities that would otherwise require extensive manual effort.
By improving data quality through AI while simultaneously needing reliable data for AI, businesses establish a virtuous cycle. Agentic AI, in particular, benefits from having consistent, verified data; without it, the likelihood of decision failures rises sharply.
Combining both approaches creates a feedback loop in which data remains continuously trustworthy, allowing AI to operate with minimal human intervention and maximum impact.
Q: Where’s the human in the loop if AI is curating the data, processing the data and giving insights from the data?
A: Even if AI is curating, processing, and deriving insights from the data, humans remain indispensable. They not only define and prioritise data quality rules but also validate AI-derived insights and resolve ambiguities AI alone cannot handle.
At Syniti, our expert consultants – each with years of business and functional experience from a data-focused lens – play a pivotal role in “joining forces” with AI. They help procure and refine the high-quality data rules and reports that are ultimately used by our customers, ensuring alignment with real-world business processes and requirements.
This blend of human expertise and AI-driven analytics keeps organisations in control, prevents AI systems from going off track, and facilitates proper handling of critical decisions.
By maintaining this human-in-the-loop approach, businesses can harness the speed and efficiency of AI while preserving essential oversight and accountability.